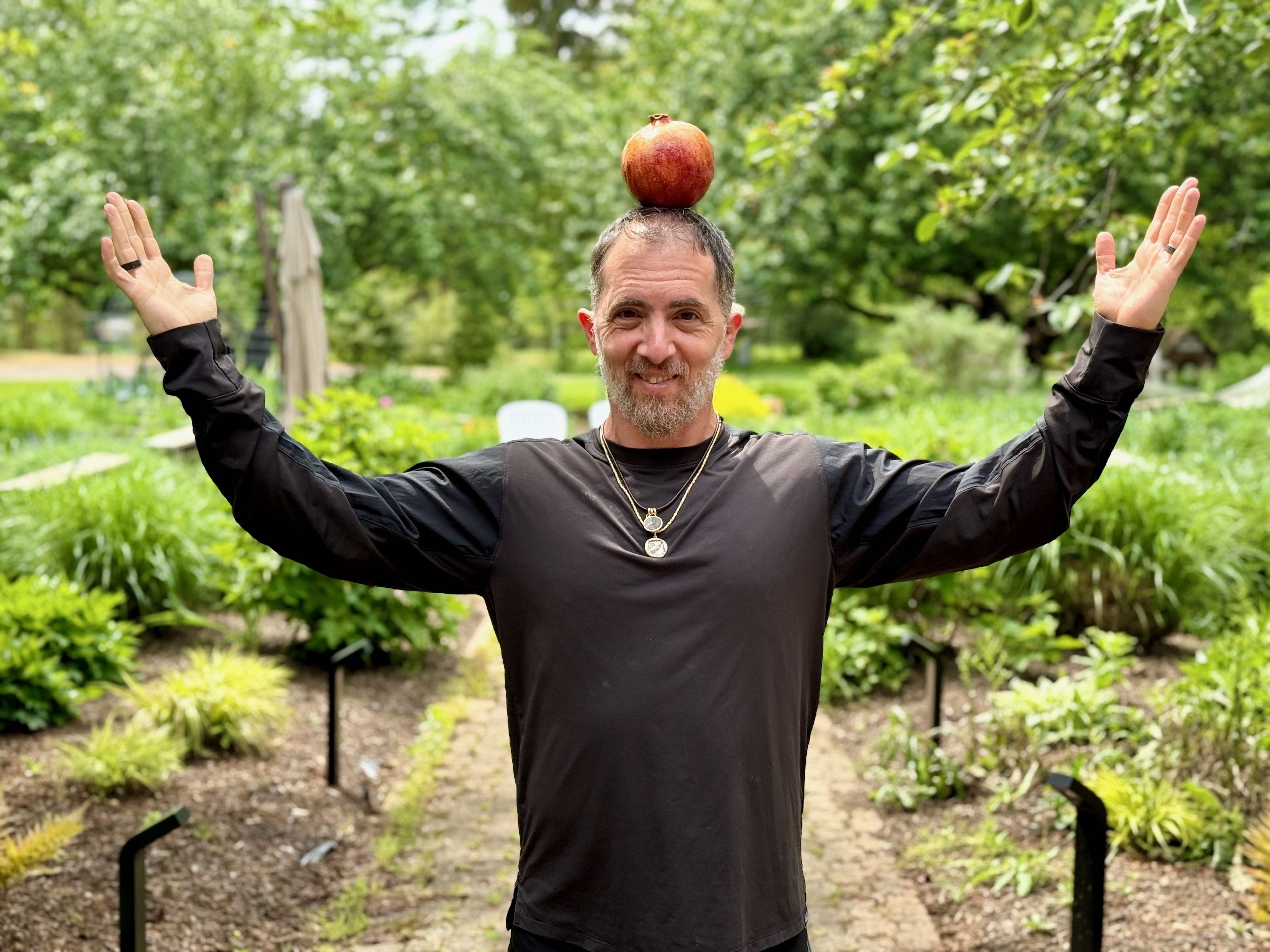
Dr. Joshua T. Vogelstein
Founding Director of the NeuroData lab since 2014
The important stuff:
For more info: blog, wiki, twitter, facebook, instagram, linkedin, scholar, github, CV, email, or hang out with me.
- 2009: PhD in Neuroscience and MSE in Applied Mathematics and Statistics from Johns Hopkins University
- 2011: Co-founded Open Connectome Project, absorbed by Applied Physics Lab in 2020
- 2015: Co-developed ML method which is Google's default tabular ML method since 2023
- 2018: Co-founded Gigantum, purchased by Nvidia in 2022
- 2019: Co-developed graph machine learning library with Microsoft Research
- 2023: Co-senior author on first brain connectome ever published, NatGeo top medical breakthrough of the year
- 2025: Co-authored monograph on graph machine learning
Founding Director of the NeuroData lab since 2014
The important stuff:
- 1983: fell in love with the love of my life
- 2014: married the love of my life
- 2017: Lion born
- 2019: Owl born
- 2022: Hawk born
For more info: blog, wiki, twitter, facebook, instagram, linkedin, scholar, github, CV, email, or hang out with me.